Common Data Problems for Marketers and Effective Solutions
- Barri Coen
- Jun 1, 2023
- 4 min read
Updated: Jun 5, 2023
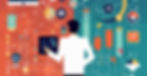
Growth Marketing and Digital Marketing are inherantly data-driven. Without good quality, accurate, accessible data, it's almost impossible to make good decisions in terms of how to grow your business.
However, marketers often encounter various challenges related to data access, management, and analysis. In this article, we will explore six common data problems faced by marketers and provide effective methods to solve each problem.
Jump straight to your issue:
Most common data problems
1. No Data Access
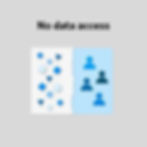
Marketers often struggle with limited or no access to relevant data, hindering their ability to gain valuable insights and make informed decisions.
Reasons for no data access:
Lack of data access can occur due to technological limitations, organisational barriers, or data privacy concerns.
Methods to Solve issues of no data access:
Data Governance and Collaboration: Establish a data governance framework to define roles, responsibilities, and data access protocols. Encourage cross-functional collaboration to ensure marketers have access to the data they need for their campaigns.
Data Partnerships: Form strategic partnerships with data providers or vendors who can supply relevant data. This can include market research firms, third-party data providers, or even data-sharing collaborations with other organisations.
Invest in Data Infrastructure: Implement robust data infrastructure and storage solutions that facilitate easy access and retrieval of data. Cloud-based platforms or data lakes can help centralise and provide secure access to data across the organisation.
2. Too Much Raw Data
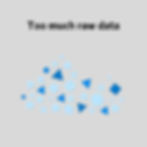
Marketers often face the challenge of handling vast amounts of raw data, making it difficult to derive meaningful insights.
Reasons for too much raw data:
The proliferation of digital channels, social media platforms, and marketing tools generates an overwhelming volume of unstructured and unrefined data.
Methods to solve issues of too much raw data:
Data Filtering and Segmentation: Define specific parameters and filters to extract relevant subsets of data. By segmenting the data based on demographics, behaviour, or other relevant attributes, marketers can focus on subsets that are most valuable to their marketing goals.
Automation and AI-Powered Analytics: Leverage automation and artificial intelligence (AI) tools to analyse large datasets efficiently. Machine learning algorithms can help marketers identify patterns, trends, and actionable insights within the data.
Data Visualisation and Reporting: Utilise data visualisation tools to present complex data sets in a visually engaging and easily understandable format. Interactive dashboards and reports allow marketers to identify trends and make data-driven decisions quickly.
3. Siloed Data

Siloed data refers to data that is isolated and fragmented across different departments or systems, making it challenging to derive a comprehensive view of customer behaviour and marketing performance.
Reasons for Siloed Data:
Siloed data often stems from a lack of data integration, legacy systems, or departmental barriers.
Methods to solve issues of Siloed Data:
Data Integration and Centralisation: Implement a robust data integration strategy to consolidate data from various sources into a centralised data warehouse or data lake. This ensures that marketers have access to a holistic view of customer data and marketing performance.
Cross-Functional Collaboration: Foster collaboration between different departments and teams to encourage data sharing and alignment. Establish processes for regular data synchronisation and communication across teams to break down data silos.
Invest in Marketing Technology Stack: Implement a marketing technology stack that includes tools for data integration, CRM systems, and marketing automation platforms. These technologies can help streamline data management and enable seamless data flow across different marketing channels.
4. Manual Data Centralisation

Manually centralising data from various sources is time-consuming, error-prone, and can delay data analysis and decision-making.
Reasons for manual data centralisation:
Lack of automation, disparate systems, or reliance on outdated processes can lead to manual data centralisation challenges.
Methods to solve manual data centralisation:
Marketing Data Management Platforms: Invest in marketing data management platforms (DMPs) that can automate the process of data centralisation and integration. DMPs enable marketers to aggregate, cleanse, and transform data from multiple sources in a centralised manner.
Application Programming Interfaces (APIs): Utilise APIs to connect different systems and automate data transfers between them. This eliminates the need for manual data entry or extraction, ensuring accurate and up-to-date data.
Data Cleaning and Standardisation: Implement data cleaning and standardisation processes to ensure consistency and accuracy across different data sources. Tools such as data cleansing software or data validation scripts can help automate these processes.
5. Scattered Data
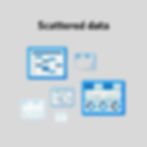
Marketers often encounter scattered data across multiple platforms, making it challenging to have a unified view of customer interactions and marketing campaigns.
Reasons for Scattered Data:
Data fragmentation can occur due to the use of disparate tools, lack of data integration, or poor data management practices.
Methods to solve Scattered Data:
Unified Marketing Analytics Platform: Implement a unified marketing analytics platform that integrates data from various marketing channels and platforms. This platform should provide a consolidated view of customer interactions, campaign performance, and marketing ROI.
Customer Data Platform (CDP): Invest in a CDP that aggregates and unifies customer data from multiple sources. A CDP enables marketers to create a single customer view and deliver personalised marketing experiences across channels.
Data Migration and Consolidation: Conduct a thorough audit of existing data sources and consolidate them into a centralised data repository. This may involve data migration, extraction, and transformation to ensure a unified data ecosystem.
6. Slow Time to Insights:

Marketers often face delays in obtaining actionable insights from data due to complex data analysis processes or inefficient data management practices.
Reasons for having a slow time to gain insights:
Inefficient data processing, lack of automation, or limited analytical capabilities can contribute to slow time to insights.
Methods to solve having slow time to insights:
Predictive Analytics and Machine Learning: Leverage predictive analytics and machine learning algorithms to automate data analysis and derive real-time insights. These technologies can help marketers identify trends, predict customer behaviour, and optimise marketing campaigns.
Data Preprocessing and Optimisation: Implement data preprocessing techniques to clean, transform, and optimise data before analysis. This includes data normalisation, feature engineering, or outlier detection to enhance the quality and relevance of data for analysis.
Agile Data Analytics: Adopt an agile approach to data analytics, focusing on iterative and incremental data analysis. Break down analysis tasks into smaller, manageable chunks to speed up insights generation and enable faster decision-making.
In Conclusion
Effective data management is crucial for marketers to drive successful marketing campaigns and make data-driven decisions.
By addressing common data problems such as limited access, overwhelming data volumes, data silos, manual processes, scattered data, and slow time to insights, marketers can unlock the full potential of their data. Implementing the suggested solutions will empower marketers to leverage data effectively and gain a competitive edge in the dynamic world of marketing.
Speak to our data experts to resolve any of the issues above.